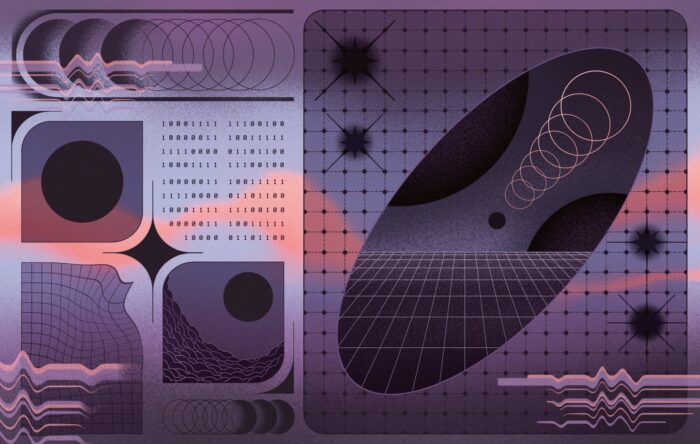
In turn, we use cookies to measure and obtain statistical data about the navigation of the users. You can configure and accept the use of the cookies, and modify your consent options, at any time.
Is Artificial Intelligence a Myth?
Exploring whether human sense-making can be surpassed by AI.
Illustration: Milos Novakovic
Erik Larson is a tech entrepreneur and pioneering research scientist working at the forefront of natural language processing. He recently published a book called The Myth of Artificial Intelligence: Why Computers Can’t Think the Way We Do. FARSIGHT met him online for an interview about the future of AI, and why he believes the field’s current path of development will not lead us to human-level intelligence in machines anytime soon.
Erik, what made you decide to write this book?
My specialty is natural language processing, and I wrote the book from the perspective of understanding the many practical challenges and difficulties there are in making computers understand human language on a deep level. Early in my career, I read a book by Ray Kurzweil, The Age of Intelligent Machines, where he proposed 2029 as the year when computers become as smart as humans. I thought, maybe – it’s 30 years, after all. By 2005, when his book The Singularity is Near came out, I thought that it could not happen in 20 years without some major unexpected scientific breakthrough that we couldn’t anticipate yet. Instead of acting like we’re on an inevitable path to general AI, we should tell the broader public that achieving true computer intelligence is a lot more difficult than many assume. That’s why I wrote the book.
You argue that we are very far from developing general artificial intelligence. In fact, you believe that the approach we are currently pursuing can never lead us there. Why is that?
The main framework that I use in the book is inference. In AI, the problem is that we’re using the wrong type of inference to ever get to general or commonsense intelligence. Right now, the field is almost exclusively dominated by machine learning using inductive inference, learning from prior examples. Human beings use induction all the time, but it’s not the most important type of inference for us. It can’t handle novelty because it’s based on prior observation. Without a novelty mechanism, you can’t get to certain kinds of intelligence. I don’t mean to say that it’s impossible. Nature has developed general intelligence, so we should be able to eventually do the same thing. However, there’s something currently missing, and that’s why it’s been so difficult to make certain kinds of progress in the field.
Arthur C. Clarke famously thought that to get something like intelligence in a computer, we would need heuristic logic – finding and using solutions that aren’t precise, but just good enough, which is how we think. We don’t measure the distance across the street with a measuring tape; we guesstimate how far it is. This method is a lot faster and works well for everyday stuff. Do you think we could program that kind of heuristic logic into computers?
We do that already. Before deep learning became the dominant paradigm in AI development, classic AI design was more rule-based. One of the great challenges in the classic rules-based paradigm was in fact to find these rules of thumb, or heuristics. Herbert Simon, a pioneer in AI and Nobel Prize winner in economics, has said that people who favour adequacy and efficiency over optimisation generally make better, more responsible, and quicker decisions than those who want to make every decision perfect. Precision can be a barrier. However, the classic AI approach based on common-sense heuristics also failed when the domain wasn’t sufficiently constrained. Even if you have a rule that doesn’t need precision, you have so much context in an unconstrained real-world environment that you need rules to tell other rules when they are relevant. It quickly becomes intractable to try to get intelligent behaviour from such a system.
There are two major, unsolved problems in AI. One is robotics, especially when the robot is not in a very specific environment. A robot arm in an industrial setting with few degrees of freedom works well, but if we have a robot walking down the street in Manhattan, there are just so many peripheral problems that can occur in such a complex environment. Somebody walks in front of the robot; something unexpected happens. If you took the best, smartest robot in the world and set it loose on any city street, within a few minutes it would cause a traffic accident. That’s why you don’t see robots on the street.
Broaden your horizons with a Futures Membership. Stay updated on key trends and developments through receiving quarterly issues of FARSIGHT, live Futures Seminars with futurists, training, and discounts on our courses.
BECOME A FUTURES MEMBERThe other major problem is having a real conversation with an AI system where it truly understands what you’re saying and responds with understanding. I mentioned inference before, and in addition to deduction and induction, there’s a third type of inference called abduction that people generally aren’t aware of, but which we use all the time. Deduction is, “It’s raining; therefore, the streets are wet.” Abduction is, “I know rain makes streets wet. I see the streets are wet. Perhaps it’s raining.” You generate a hypothesis that explains an observation. It’s not certain knowledge – you could be wrong. Maybe a fire hydrant broke. However, you keep correcting your hypothesis with further observation. The streets are wet, my hypothesis is that it’s raining, and then I confirm it or form another. That’s abduction – hypothesis generation.
You mentioned novelty. A human who has not been in a certain situation before can think it through and still handle it. If you introduce a chess master to Shogi, Japanese chess, which has slightly different rules, they would very quickly be able to adapt their experience with chess to be able to play it well. A chess-playing AI, however, would have to learn from scratch – its inductive deep learning of chess would be useless.
I believe game-playing AIs still use some version of a min-max algorithm, deducing what would be the best move given that it has watched a million games play out before. This is very different from a human, who doesn’t play a million games and then computes the probability. I’m not a neuroscientist, so I couldn’t tell you what’s happening in the brain of chess masters – but I’m pretty sure they don’t mindlessly play a million games before becoming masters.
I’ve observed that as computers get better than us at something, like chess or trivia knowledge, we tend to move the goalpost and say that this has nothing to do with intelligence. Will we keep redefining intelligence as being whatever we can do that computers can’t, or are there some markers of intelligence that we can’t explain away?
My response is to go back to Alan Turing’s original 1950 paper, when he said that if a person can converse with a computer and be convinced that it is a real human, then it must be intelligent. I would say that this test still holds. Of course, you can converse with a chatbot that just continues to deflect questions, but to have a conversation that’s empathetic and understanding with the computer – we still can’t do that.
During the summer of 2022, a big news story surfaced of a Google engineer becoming convinced that a program he was developing had gained real sentience and warranted rights akin to human rights. Could we not say that it passed the Turing test?
The latest language models are quite good, but you can trip them up very easily if you know how. Language has a property called compositionality, how sentences are put together to provide meaning. There’s a big difference between me riding a horse and a horse riding me, but an AI language model is not going to get that because it doesn’t have a sense of compositionality. Natural language is a barrier for artificial intelligence – one of the biggest. A legitimate test of language understanding would convince me that an AI was intelligent.
Another test would be navigation in dynamic environments by autonomous vehicles or robots. Getting to fully autonomous driving will be a lot harder than people think. The small city of Palo Alto, California, is mapped out on a grid, and you get pretty good performance from the vehicles there. But if you’re driving on a rural road and the AI must rely on sensor data, we’re a long way from vehicles being able to autonomously navigate that. Fully capable robotics in openended dynamic environments and fully understanding natural language; those are the two big frontiers.
Could an AI not develop its own language, very different from human language, that it uses to understand its environment and gets around some of the current limitations? We could compare it to communicating with dolphins, which seem to have a complex language that we haven’t come close to understanding. They cannot understand our questions, and we cannot understand theirs; yet they are doubtless sentient beings.
I suppose is it possible for a creative AI to somehow achieve a way to frame the world that doesn’t require natural language. I don’t know the answer to that, but the immediate practical problem I see is how do we then interact with those systems? That might create some very, very strange human-machine interactions. I almost completely avoided the question of sentience in my book because, frankly, I don’t have a lot to say about it. It’s an issue that very quickly becomes philosophical. It could be that computers right now have some low level of sentience, like insects, and we just can’t detect it because we don’t know how. As an engineer, I don’t know the entry point into that argument, so I leave it alone.
You argue that we can’t achieve general AI the way we try to do it now, with machine learning and adding more components to computers. However, in physics there’s the phenomenon of ‘emergence’, where new traits develop when the complexity is high enough. One water molecule doesn’t have surface tension, but put enough together, and you get it. A single neuron isn’t sentient, but enough produce human sentience. Would it not be possible, if we add complexity and more components to supercomputers, that they could achieve intelligence and sentience as an emergent trait?
I think it’s an interesting question. It’s like a pile of sand: if you keep adding grains of sand, you get a nice conical shape, until at one point adding just one more grain of sand gets you a cascading effect. We have these thresholds in emergence where something isn’t happening, and then at some level of complexity, a completely different phenomenon emerges. I think it’s interesting whether that could apply to technology or computers, but I don’t have any strong scientific position on that.
Isn’t there a danger if we have, say, self-driving cars who all think the same way because we have copied the same machine learning into all of them? If there are several routes from a suburb to the city, they will all choose the same route because that’s what the system says they should do, whereas humans might imagine that the main route will probably be too busy and choose another one instead?
I think we’ll solve those sorts of problems. We already have systems where you can see traffic flow. The problems that I worry about are more practical. There have been cases where self-driving cars don’t stop because a stop sign is slightly damaged and is perceived as something else. There’s a famous example of a system
that tried to drive underneath a school bus because it thought it was an overpass. We just can’t eliminate all problems because the natural world is so messy. A bunch of leaves that the wind blows across the street might be interpreted as a solid object, and the AI will slam on the brakes.
We have people worrying that if we achieve general intelligence in computers, they are going to take over the world, or follow some order, like maximising the production of paperclips, to such extremes that the AI will wipe out humanity to do it most efficiently. Do you think there is any real danger of such things happening, or are we just projecting our own faults onto artificial intelligence?
There’s an interesting contradiction in the paperclip scenario. The system is supposed to have general intelligence, which you would think included common sense, but on the other hand, it’s so narrow and computational that it thinks it can maximise the sale of paperclips by turning all humans into paperclips. Real computer intelligence would realise that it’s not intended to wipe us out. There’s another option, though, which is that it becomes malevolent and actively desires to rid the world of human beings. That gets us into the question of whether something like malevolence could possibly emerge in an AI.
We have AIs today that looks at x-rays of patients, trying to determine if they have cancer. They can be very good at this, but they don’t know anything about cancer or what it means to a human being. They lack an understanding of what their task really is about. Do you think we can achieve intelligence in computers without true understanding of what they do?
That’s a great question, but I don’t have a great answer for it. It raises the whole issue, in this case of medical science, of whether an AI can provide proper diagnoses when it doesn’t understand care. Someone should write a PhD about how medicine is best administered and what the role of technology is and can be.
Research shows that even when an AI is better than any doctor at diagnosing cancer, it is even more efficient when it works with a human doctor. They approach the problem in different ways – one with human understanding, the other from being trained on millions of x-rays. Human-AI partnerships seem to work best.
I think that’s right. In terms of something we care about, like medicine, it sounds like this kind of collaboration may work best. To me that’s a good use of technology. That’s why we make technology – because it furthers human goals. Whether we will have autonomous systems that will replace humans in all domains, that is a completely different question. Whether we get fully sentient AI or not, we’re heading in this direction in the future. That’s for sure.”