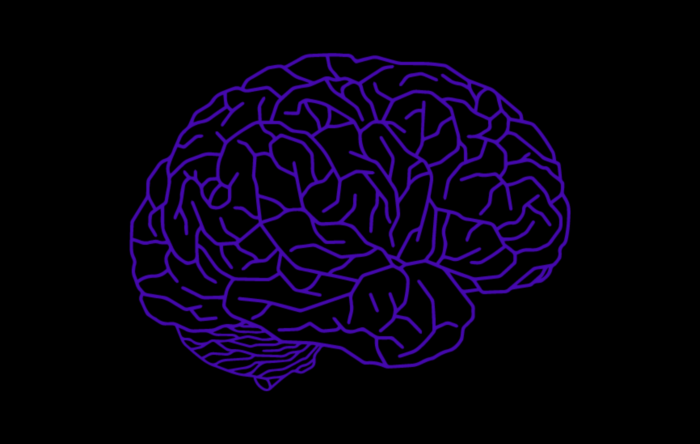
In turn, we use cookies to measure and obtain statistical data about the navigation of the users. You can configure and accept the use of the cookies, and modify your consent options, at any time.
Falling Through The Cracks
Cataloguing Gender and Sex in Electronic Health Records
Electronic Health Records (EHRs) are used widely in the healthcare sector to systematically organise patient data in a digitised format. And in an increasingly digitised healthcare industry, they are critical in allowing providers to easily share patient data across laboratories and specialists. However, the binary nature of EHRs – which is what allows for streamlined data sharing and making these datasets lucrative for training machine learning algorithms – also present challenges when classifying demographic information of patients, such as a patients’ sex/gender.
We sat down with Maggie Delano, Professor of Engineering at Swarthmore College, to talk about their research on how EHRs capture sex/gender in binary categories, the clinical implications of when a patient does not fit within the system, and the integral role of inclusive design in improving these digital health technologies.
Can you tell me a little bit about how EHRs have categorised sex/gender historically? How did we get here?
Historically, there was not really distinction between sex and gender. And although there was a lot of disagreement about what sex actually meant as far back as the late 1800s, by the time we had electronic health records, the idea was that sex was male or female. And so that’s the only information that was incorporated into the Electronic Health Record.
Broaden your horizons with a Futures Membership. Stay updated on key trends and developments through receiving quarterly issues of FARSIGHT, live Futures Seminars with futurists, training, and discounts on our courses.
BECOME A FUTURES MEMBERThis information was used to make certain screening decisions easier. At least in the US, it was also used for insurance purposes to figure out reimbursement for certain procedures. For example, if they can assume female patients don’t have testicles, they can filter out what kinds of procedures should apply to someone. EHRs are also used for reference information – if you take a blood test, there could be sex-based differences – so they use the sex category as reference information.
What does this mean for patients whose gender does not match their assigned sex at birth? Does this conflation between sex and gender ever become an issue?
I think in recent years, there’s been a lot more acceptance of transgender and intersex people. This is leading to an increased awareness of the sex variable – male or female – and how it is causing issues because it doesn’t capture the full experience of a patient. This is true even for a cisgender patient. For example, if a person has had a mastectomy and they are identified as female in the EHR, notifying them that they should have a breast exam may not actually be relevant. There’s a lot of research that indicates if you’ve had a double mastectomy, then your risk of breast cancer is very low because most of your breast tissue has been removed.
But especially for transgender people, things get complicated because there are certain times when it makes sense to follow your assigned sex at birth. If you’re transgender but you haven’t started taking exogenous hormones, then it makes sense to continue following a lot of these screening procedures based on assigned sex at birth. On the other hand, once you have had certain procedures, it could make more sense to follow screening procedures based on gender. What’s difficult is that there’s not a lot of research indicating when which one – sex or gender – is most appropriate.
How does this play out in a clinical setting?
A lot of doctors that are working with EHR companies have basically said, “We think the sex that was assigned at birth matters more than what the person identifies as now.” So, they added this gender category to improve patient care in the sense of not misgendering a patient. But really, it was so they could avoid having to update a lot of the reference ranges, screening software, etc.
There’s this perception that we’re moving towards a scenario where things are much more inclusive. However, the gender field isn’t substantively changing clinical care, at least not the way that it’s implemented now. It leaves it up to the patient and their individual provider to figure out what treatment makes the most sense. And in some cases, that is not necessarily a bad thing. But it does mean that this reliance on sex assigned at birth, and the assumption that sex is the most relevant category, creates issues. Without a lot of further research, we can’t know what kind of treatments are appropriate for what kinds of people.
This has implications for big data and machine learning. Because even though the gender category is slowly being implemented into EHRs , a lot of these datasets don’t include that information at all. Therefore, any transgender or intersex patient is lost in the noise. There’s so much more that we could be doing to support those patients and to also understand the science better. Clinical medicine cannot rely on this one variable to stand in for so many different clinical parameters.
EHR data are especially appealing for machine learning purposes. In your research, you illustrate how EHR data are used in both natural language processing and by using previously collected data. How do you see either of these uses of sex/gender categories carried out in research, and what are the dangers?
When these data are used in a machine learning algorithm, they’re assumed to be binary values. In reality, that doesn’t capture the full range of underlying variables that might be related to sex and gender. Someone may be assigned female at birth, but that doesn’t mean they have all these anatomical features that we presume to be tied to one’s sex. So right now, we automatically assume a lot from the sex category.
What if instead, we asked, for the research question at hand: which of the variables are we really interested in? And could we get those variables instead? One of the challenges is the concern that there would be too many variables. But in a lot of cases, those variables are available, they’re just not included in the model. Some of the machine learning papers that we reviewed had 30 or even 50 clinical variables, but they just had one or two for demographic information. There was a lot more that they could have been looking at, that they just didn’t.
There are also intelligent ways that EHR systems could be designed to have smart defaults. For example, there’s a paper from a nursing journal that we cite in our research that talks about how there are ways that you can design user interfaces so that if the patient is cisgender, you can intelligently fill in a lot of defaults. Then, if any patient is different from that default, you can easily change it. In this way, you’re not necessarily increasing the administrative burden, but you’re making it much easier to input that information.
You argue for sex and gender to be considered contextually. And in your paper, too, you talk about it being conceptualised more like a family history field. Can you elaborate on what you mean by that?
We were inspired by a paper that used diabetes as an example. One of the things they found was that in a lot of studies, patients are forced to answer yes or no to whether they have diabetes. It’s easy to program and to understand, but what we really need to know are things like, what kind of diabetes? When was the onset of diabetes? How long has the patient had diabetes? Having that additional context allows you to have a more holistic picture. Even researchers who are thoughtful about the origins of their data can make the mistake of thinking that sex is one of the things in a dataset that does not need further unpacking. That’s what we mean when we say sex should be related to family history; sex is more like ‘‘family history’’ than it is like ‘‘age.’’
When you think about it in a machine learning context, including these additional variables has the potential to make for a much more useful, predictive algorithm. It depends on what the goal of the algorithm is, but it could increase the ability to do something useful, rather than drawing these vague trends that don’t really fit anyone or anything.
What do you think is necessary to untangle the sex/gender category in future research?
I think of these variables as a kind of tightrope. Some people assume that sex matters, or that there’s a significant sex difference, and therefore you need to know someone’s sex assigned at birth. Then there’s the other argument that sex isn’t relevant at all.
The issue is that we don’t know what we’re dealing with when we’re dealing with sex. And so, I think a lot of the future research needs to look into finding a way to walk the tightrope between assuming that sex matters, and assuming that it doesn’t matter at all, to get a finer resolution.
There’s a lot of focus on sex differences in women’s health, which is where the interest in sex and gender has historically come from. However, the field has also historically been trans-exclusionary because it holds that since women’s health is different from men’s health, sex differences are a foregone conclusion. There are circumstances where that’s clearly true but there are other circumstances where that’s not true.
One of the greatest benefits of this increased focus on women’s health has been the ability to disentangle some of the complexities. For example, there are sex differences in heart attack presentation, but that doesn’t mean that women present entirely different symptoms than men. It’s that there are certain symptoms that are more prevalent in women, but that occur in men too. It’s this kind of unravelling which improves the understanding of the field.
Technological innovations such as EHRs and access to aggregated patient data can create new avenues for efficiency and ease but also, as we’ve discussed, the potential for harm in our healthcare systems especially for marginalised individuals. Reflecting on this, what is one meaningful intervention that you see as the way forward in minimising harm and maximising the benefits of EHRs?
I think that the field is somewhat stuck. And we’re trying to get them to move in a different direction. Increasingly, papers are coming out that show a willingness to acknowledge that there’s a problem with only having sex in the dataset and admitting that they don’t know the gender of a patient. Or, that their dataset can’t engage with non-binary or trans people, because they just don’t have that information.
There needs to be more incentives and venues for clinicians and researchers to get credit for doing this work, because there’s not enough funding to study these kinds of things. Even when researchers do find a way, there aren’t always venues for them to share that work. So there’s also a need for carving out the space and supporting the people who are already doing the work.
You work with a concept called Design from the Margins, which is a design process that centres the most marginalised groups. How does this approach benefit trans and non-binary people, but also cisgender people, when working with EHRs?
The Design from the Margins framework comes from a report by Afsaneh Rigot, a researcher who covers law, technology, LGBTQ, refugee, and human rights issues. There’s a great bell curve figure in Afsaneh’s paper illustrating that when you design for the margins, you start from the outside and it covers everyone inside by default. So rather than thinking of this as designing for these tiny slivers on either end of the bell curve, it’s designing from that point inward. That’s the key intervention: if you design from outwards in, everyone inside is automatically included.
It goes back to what I mentioned earlier about the female patient who had the double mastectomy. That person could be cisgender or trans, and the recommendations would be similar, assuming other variables were not so different. One of the things that we were thinking about when writing the paper was also that designing for the margins wouldn’t necessarily be as difficult as people perceive it to be. But also, even if it is difficult, there are clear benefits from doing it for everyone. The transgender and intersex people are just the canary in the coal mine. They’re the example of why this is failing, it’s just failing for them in a more catastrophically obvious way than it is for other people.
CHECK-UP is a Q&A series by Maya Ellen Hertz and Sarah Frosh exploring advancements and providing critical reflections on innovations in digital health. From telemedicine and electronic health records to wearables and data privacy concerns, the article series includes interviews with experts in fields across law, engineering, and NGOs who shed light on the myriad of complexities that must be considered in the wake of new digital health technologies.